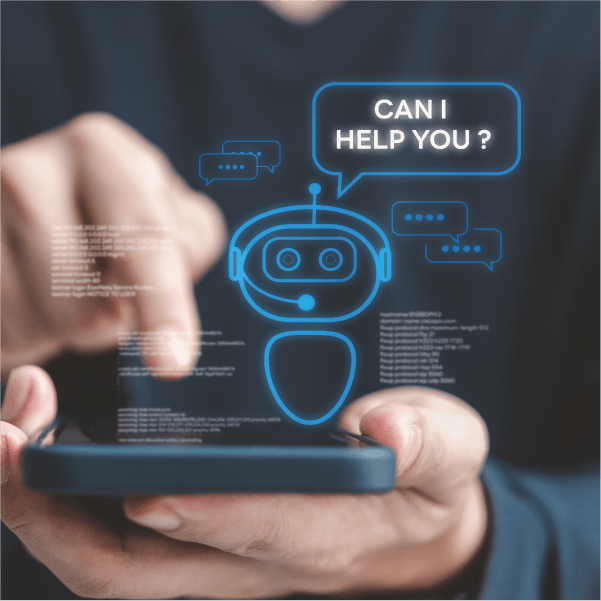
Tracking Sales Processes Efficiently with AI-Enabled Chat Bot:
Explore how an AI-driven chatbot leverages Outlook emails to enhance communication and efficiency within sales teams. The system preprocesses emails and stores them in a database, then reconstructs the most relevant email conversations in response to natural language queries, simplifying the tracking of the sales process.